Multi-channel input noise removal technology to overcome limitations of existing noise removal technologies adaptively and concurrently removes static and dynamic noises using differences between microphone input signals. Independent vector analysis-based technology contributes to upgrading voice recognition interface performance in real living environments where various types of noises exist, such as from TV, radio and from people conversing.
Key algorithm
Independent vector analysis (IVA) technology solves the problem of permutation in the orders of frequency properties by inducing learning rules to minimize mutually common information volume using a probability distribution model. For this, it takes into consideration the dependence between frequency properties. Therefore, this technology can separate target voice from unknown ambient noise.
- IE Soft-mask: As a result of IVA controllability, reliability of the output signal increases. In addition, it only involves the process of interference extraction, which is different from existing processes. As it does not require target tracking, it ensures that a more concise algorithm is obtained.
- IVA is a blind source separation algorithm to separate independent outputs of the same number using a number of observation signals. It is run through a method to minimize cost function, which measures mutual information dependence between each output. When a result to optimize separation matrix is derived in relation to the cost function, mutual statistical independence of the two output signals is maximized. In particular, to define the score function used, IVA models higher-level dependence between frequency properties of the same sound source signal by assuming multivariate super-Gaussian distribution for preliminary probability information of the sound source. Therefore, the problem of permutation, which occurs as the orders of sound sources are jumbled when frequency domain signal is restored to a time domain signal, is solved. In addition, it produces superior separation performance to the existing algorithms.
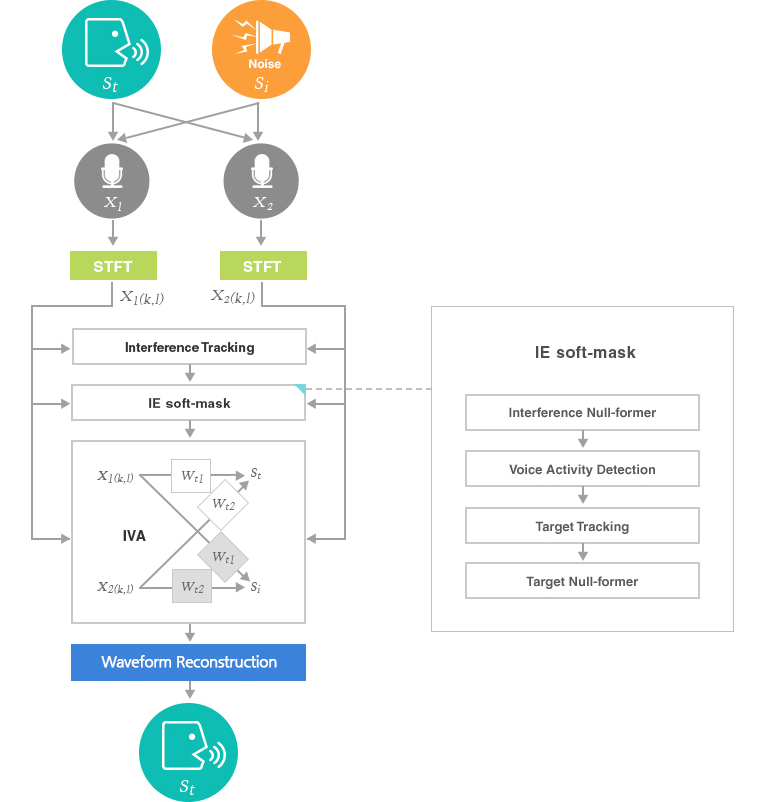